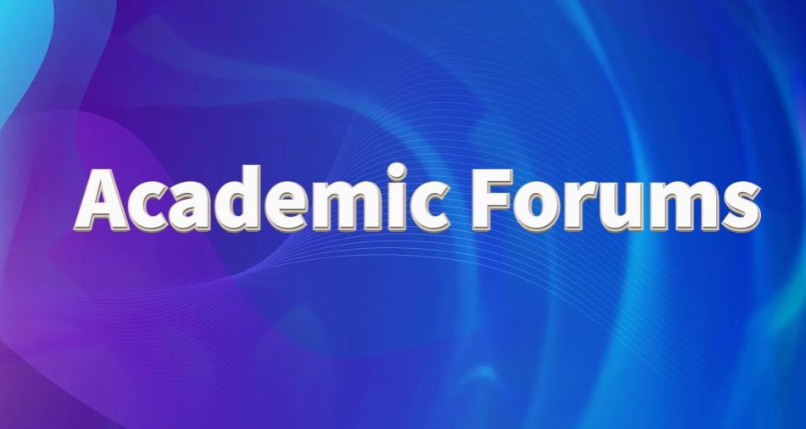
Report Time: 14:30 - 16:00, November 13, 2024
Tencent Meeting ID: 688 - 930 - 339
Host: Feng Zhiguang
Wang Shuqiang
Researcher, Shenzhen Institute of Advanced Technology, Chinese Academy of Sciences
Research Field: Medical Image Computing
Wang Shuqiang is a specially - appointed researcher of the Chinese Academy of Sciences, a supervisor of doctoral students at the University of Chinese Academy of Sciences, and the deputy director of the Biomedical Information Center of Shenzhen Institute of Advanced Technology, Chinese Academy of Sciences. He is mainly engaged in the research of medical artificial intelligence, brain image - brain network computing and other fields. As the person in charge, he has presided over more than 20 projects, including key R & D projects of the Ministry of Science and Technology, 4 projects of the National Natural Science Foundation of China, the Jiangsu Provincial Outstanding Young Scientists Fund, and key projects of Shenzhen. He has been authorized more than 40 invention patents, including 6 US invention patents. He has published more than 150 papers in international journals such as IEEE TPAMI/TMI/TIP/TNNLS/TSMC/TSP/TII/TNSRE/TASE/TAI,
Medical Image Analysis and international conferences such as ICLR, IJCAI, AAAI, MICCAI. His H - index is 42. He has been selected into the annual scientific influence list and the lifetime scientific influence list of "Top 2% Global Scientists" of Stanford University for many times. He serves as an associate editor of SCI journals such as
IEEE Transactions on Consumer Electronics,
Brain Informatics,
Frontiers in Neuroscience.
The rapid development of brain imaging technology has provided great convenience for non - invasive analysis of brain structure and function. Brain networks can help people better understand the occurrence mechanism of nervous system diseases. Brain image - brain network computing is an important tool and means for analyzing brain cognitive mechanisms and predicting brain diseases. Generative AI algorithm models represented by VAE, GAN, and Diffusion have broad application prospects in brain image - brain network computing.
This report first shares the work on cross - modal reconstruction and 3D brain reconstruction of brain images based on generative AI algorithms such as GAN and VAE. It further explores the main problems and challenges faced by brain image - brain network reconstruction, and analyzes the advantages and disadvantages of existing brain network construction tools. Finally, aiming at the challenges of heterogeneity and heterogeneity in the process of multimodal brain image fusion, a multimodal brain network computing method based on brain structure - function fusion representation learning is proposed. Taking Alzheimer's disease as an example, the changes in abnormal brain connections at each stage are analyzed and compared. The experimental results show that the related models are expected to provide potential biomarkers for early AD prediction.
College of Intelligent Systems Science and Engineering, Harbin Engineering University